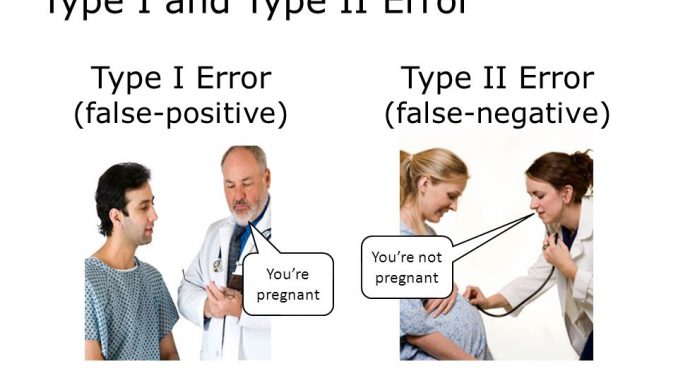
Table of Contents
What is Hypothesis Testing?
- Hypothesis testing is a statistical test used to determine the relationship between two data sets, between two or more independent and dependent variables.
- Hypothesis testing is a scientific process to conclude whether to reject or not reject the null hypothesis.
- Statistical methods are used for hypothesis testing. Thus, hypothesis testing is also known as statistical hypothesis testing.
What are the Steps of Hypothesis Testing?
Hypothesis testing consists of four steps. The steps of hypothesis testing are:
1. State hypothesis:
a) State Null hypothesis:-
-
- Null hypothesis is a statement which states no relationship between two variables or two phenomena.
- E.g of stating null hypothesis: There is no significant relationship between smoking and lung cancer)
- Null hypothesis is usually denoted by H0.
b) State an alternative hypothesis/alternate hypothesis:-
-
- Alternative hypothesis/alternate hypothesis is a statement which states some statistical significance between two phenomena.
- E.g. of stating alternative hypothesis: There is significant relationship between smoking and lung cancer
- Alternative hypothesis is usually denoted by H1 or HA.
2. Formulate an analysis plan including determination of significance level
- To find out if the hypothesis is true or not, analysis using different methods (chi-square test, regression models etc.) are used.
- The relationship is tested at specific significance level.
- These significance level is usually taken (marked) at 5%. Also, the significance level is taken at 1%, 10% or in accordance to the confidence interval.
- The taken significance level also represents the error in the study.
- Higher the significance level, lower is the confidence level of the study and lower the significance level, higher is the confidence level of the study
3. Analyze sample data and calculate the p-value, Odds Ratio and Confidence Interval
- The statistical significance of a test is measured after formulation of a hypothesis.
- The statistical significance is determined on the basis of p value, odds ratio and confidence interval of the study.
- Different statistical tests (chi-square, regression models etc.) are used to determine the significance of the tests.
4. Interpret results
What is the Importance of Hypothesis Testing:
- A hypothesis test/research hypothesis test evaluates two mutually exclusive statement about a population to determine which statement is supported by the sample data.
- According to the San Jose State University Statistics Department, Hypothesis test is one of the most important concepts in statistics because it is how we decide if something really happened, or if certain treatments have positive effects, or if groups differ from each other or if one variable predicts another.
- Hypothesis test/significance testing of a hypothesis is a way to evaluate the plausibility of a hypothesis by using sample data.
- It is used to test an assumption regarding the population parameter.
Characteristics of a Good Hypothesis:
A hypothesis can be good if it is based on a good research question. The characteristics of a good hypothesis (Hulley et al., 2001) are :
1. Hypothesis should be simple: Hypothesis should usually contain one predictor and one outcome variable.
2. Hypothesis should be specific: It should clearly specify the subjects and variables of the study. Additionally, the hypothesis should also mention how the test of statistical significance will be applied.
3. Hypothesis should be stated in advance: Hypothesis should be stated clearly during the time of proposal development.
What do you Mean by Errors/statistical errors in Hypothesis?
In the process of testing hypotheses, there are two major types of statistical error. They are:
- Type I error/type 1 error
- Type II error/Type 2 error
What are the Differences Between Type I and Type II Error:
Basis of Difference | Type I Error | Type II Error |
Occurrence | A type I error occurs when the null hypothesis is true but is rejected. In other words, if a true null hypothesis is incorrectly rejected, type I error occurs. | A type II error occurs when the null hypothesis is false but invalidly fails to be rejected. In other words, failure to reject a false null hypothesis results in type II error. |
Comparison | A type I error also known as False positive. | A type II error also known as False negative. It is also known as false null hypothesis. |
Designation | The probability that we will make a type I error is designated ‘α’ (alpha). Therefore, type I error is also known as alpha error | Probability that we will make a type II error is designated ‘β’ (beta). Therefore, type II error is also known as beta error. |
Probability of committing error
|
Type I error equals to the level of significance (α)
‘α’ is the so-called p-value. |
Type II error equals to the statistical power of a test.
The probability 1- ‘β’ is called the statistical power of the study. |
Represents | Type I error represents ‘a false hit’. | Type II error represents ‘a miss’. |
Nature | We may reject the null hypothesis when the null hypothesis is true is known as Type I error. | We may accept the null hypothesis, when in fact null hypothesis is not true is known as Type II error. |
Importance | Type I errors are generally considered more serious. | Type II errors are given less preference. |
Acceptance | It refers to non-acceptance of hypothesis, which ought to be accepted. | It refers to the acceptance of hypothesis, which ought to be rejected. |
Consequence | The probability of Type I error reduces with lower values of (α) since the lower value makes it difficult to reject null hypothesis. | The probability of Type II error reduces with higher values of (α) since the higher value makes it easier to reject the null hypothesis.
|
References and For More Information:
Hulley S. B, Cummings S. R, Browner W. S, Grady D, Hearst N, Newman T. B. 2nd ed. Philadelphia: Lippincott Williams and Wilkins; 2001. Getting ready to estimate sample size: Hypothesis and underlying principles In: Designing Clinical Research-An epidemiologic approach; pp. 51–63.
http://www.biostathandbook.com/hypothesistesting.html
https://stattrek.com/hypothesis-test/hypothesis-testing.aspx
https://www.statisticssolutions.com/hypothesis-testing/
https://www.statisticshowto.com/probability-and-statistics/hypothesis-testing/
https://mathworld.wolfram.com/HypothesisTesting.html
https://www.simplypsychology.org/type_I_and_type_II_errors.html
https://www.bmj.com/about-bmj/resources-readers/publications/statistics-square-one/5-differences-between-means-type-i-an
https://www.abtasty.com/blog/type-1-and-type-2-errors/
https://www.thoughtco.com/difference-between-type-i-and-type-ii-errors-3126414
https://support.minitab.com/en-us/minitab/19/help-and-how-to/statistics/basic-statistics/supporting-topics/basics/type-i-and-type-ii-error/