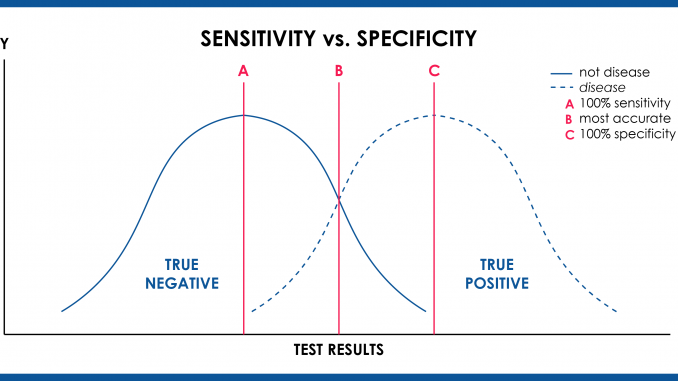
Table of Contents
What is Sensitivity of a Test?
- Sensitivity of a test (also called the true positive rate) is defined as the proportion of diseased people who were correctly identified as “Positive” by the test.
- Sensitivity of test is recognized by how good was the test that correctly identifies those who had the disease.
- In further arguments, a highly sensitive test is one that acceptably recognizes patients with a disease.
- A test that is 100% sensitive will detect entire patients who have the disease. It is exceptionally uncommon that any clinical test is 100% sensitive. A test with 90% sensitivity will detect 90% of patients who have the disease, however, will miss 10% of patients who are suffering from the disease.
- A highly sensitive test could be beneficial for presiding out disease if a person has a negative outcome.
What is Specificity of a Test?
- The specificity of a test (also called the True Negative Rate) is the proportion of non-diseased people who are correctly identified as “negative” by the test
- Specificity of test is recognized by how good was the test that correctly identifies those who did not have the disease.
- In further arguments, the specificity of a test mentions to how well a test detects patients who are not in a disease condition.
- A test that has 100% specificity will detect 100% of patients who are not suffering from the disease. A test that is 90% specific will detect 90% of patients who lack disease, however will miss 10% of patients to identify them as disease free.
- Tests with high specificity (a high true negative rate) are utmost beneficial when the outcome is positive. A highly specific test can be convenient for presiding in patients who have an assured disease.
Differences between Sensitivity and Specificity with Examples:
Sensitivity |
Specificity |
The sensitivity of a clinical test denotes to the capacity of the test to correctly identify those patients with the disease. | The specificity of a clinical test denotes to the capacity of the test to correctly identify those without the disease. |
A test with 100% sensitivity accurately categorizes all patients with the disease. | A test with 100% specificity accurately detects all patients without the disease. |
Sensitivity measure is used to define the proportion of real positive cases which got expected appropriately | Specificity measure is used to define the proportion of real negative cases, which were expected appropriately. |
Statistically, sensitivity can be calculated as
Sensitivity = (True Positive)/(True Positive + False Negative)
|
Statistically, specificity can be calculated as
Specificity = (True Negative)/(True Negative + False Positive) |
For example, a test that acceptably recognizes all positive samples in a section is very sensitive. Alternative test that merely spots 60 % of the positive samples in the panel would believe to have lower sensitivity as per it is misplaced positives and giving higher a false negative rate (FNR). | For example, a test that detects all healthy people as being negative for a certain illness is very specific. Alternative test that incorrectly recognizes 30% of healthy people as having the condition would believe to be less specific, consuming a higher false positive rate (FPR). |
The sensitivity of a diagnostic test is articulated as the likelihood (as a percentage) that a sample tests positive specified that the patient has the disease. | The specificity of a test is articulated as the likelihood (as a percentage) that a test returns a negative result specified that the patient lack disease. |
The following equation is used to compute a test’s sensitivity:
Sensitivity = Number of true positives/Total number of individuals with the illness i.e. Sensitivity = Number of true positives/(Number of true positives + Number of false negatives) |
The following equation is used to compute a test’s specificity:
Specificity = Number of true negatives/Total number of individuals without the illness i.e. Specificity = Number of true negatives/(Number of true negatives + Number of false positives)
|
High sensitivity of test result in large number of people testing positive which means more number of people who really have the disease (true positives) and also people who do not have the disease (false positive) | High specificity of test result in large number of people testing negative which means more number of people who really do not have the disease (true negatives) and also people who do not have the disease (false negative) |
In some cases, if the sensitivity of a test is high (large people screened to be positive), there seem to be:
– a burden on health systems – anxiety and worrying situation for people who are tested positive |
In case of higher specificity, a number of false negative cases also appear because of which the disease could be serious in the later stages. For eg. cancer. |
The abbreviation broadly used is SnNout (high Sensitivity, Negative result = rule out). | The abbreviation is SPin (high Specificity, rule in). |
References and For More Information:
https://www.ncbi.nlm.nih.gov/pmc/articles/PMC2636062/
https://www.statisticshowto.datasciencecentral.com/sensitivity-vs-specificity-statistics/
Leon Gordis, Epidemiology, Fifth Edition, https://ak.sbmu.ac.ir/uploads/epidemiology_gordis_5_edi.pdf
https://vitalflux.com/ml-metrics-sensitivity-vs-specificity-difference/
https://www.technologynetworks.com/analysis/articles/sensitivity-vs-specificity-318222
http://getthediagnosis.org/definitions.html
https://www.ncbi.nlm.nih.gov/pubmed/8992938
https://academic.oup.com/bjaed/article/8/6/221/406440